Resource > Damage Detection Using Large-Scale Covariance Matrix
Damage Detection Using Large-Scale Covariance Matrix
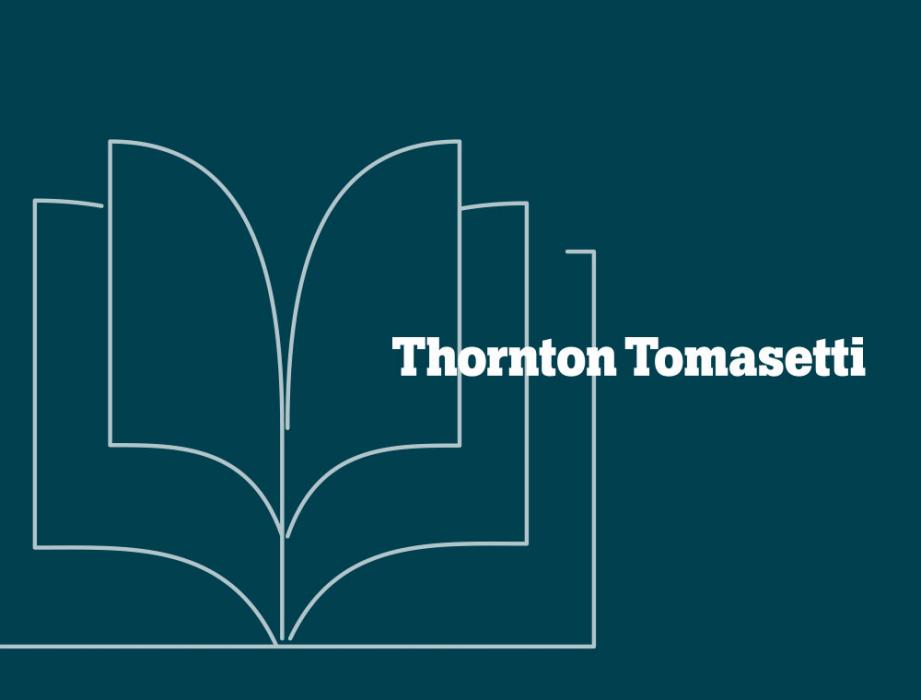
Authors
Publication
Proceedings of the 32nd International Modal Analysis Conference, Orlando, Florida.
Abstract
Statistical pattern recognition based structural damage detection is often developed exploiting the methods of outlier analysis. In this context, damage occurrence is assessed by analyzing whether a set of features extracted from the response of the system under unknown conditions departs from the population of features extracted from the response of the healthy system. The metric dominantly used for this purpose is the Mahalanobis Squared Distance (MSD). Evaluation of MSD of a point from a population requires the use of the inverse of the population’s covariance matrix. It is known that when the feature dimensions are comparable or larger than the number of observations, the covariance matrix is ill-conditioned and numerically problematic to invert in the former case, while singular and not even invertible in the latter. To overcome this difficulty, three alternatives to the canonical damage detection procedure are investigated: data compression through Discrete Cosine Transform, use of pseudo-inverse of the covariance matrix, and use of shrinkage estimate of the covariance matrix. The performance of the three methods is compared using the experimental data recorded on a four story steel frame excited at the base by means of the shaking table available at the Carleton Laboratory at Columbia University.
Keywords
Damage detection, Large-scale covariance matrix, Discrete cosine transform, Pseudo inverse, Shrinkage covariance matrix